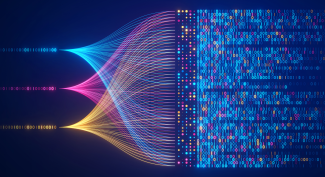
AI, Economies and Markets – How artificial intelligence will transform the global economy
Jump to a section:
AI and the global economic order
Blowing bubbles? AI's financial market implications
Introduction
In a recent survey, almost 80% of our clients told us artificial intelligence (AI) would have a transformative effect on the global economy. But the same survey revealed uncertainties as to what this transformation will look like. Clients were split on whether the AI revolution will be primarily confined to the US. And while over half thought that an AI bubble was forming in financial markets, one third were unsure.
It has been clear to us that the global debate around AI’s consequences has been missing a comprehensive and coherent framework for thinking through all of the ways that it will shape economies and financial markets. Our Spotlight report fills this gap. Below you’ll find insight into how AI will reshape the global economy from key angles, including why we expect a sizeable productivity boost in some major economies.
And, with our new proprietary AI Economic Impact Index, Capital Economics clients get a clear picture of AI’s consequences for the global economic order, including the countries that are best placed to exploit the economic benefits of AI and those that will struggle. Finally, we tie all of this together and explain AI’s implications for financial markets. Our findings are striking. We show how the AI revolution has the potential to transform the growth outlook – but also how some economies are set to benefit far more than others. We show that fears of a surge in “technological unemployment” are overdone – but also how the AI revolution will bring huge dislocation to labour markets. And we show how AI has the potential to drive a secular bull market in equities – but at the risk of inflating bubbles.
Most importantly of all, we show how AI is something that all investors need to prepare for. Beyond the considerable current hype around AI lies the inescapable fact that this technology will eventually bring fundamental and sweeping change to companies, to economies, and to the policies that regulate them.
This is by no means the last word on AI’s implications for economies and markets. Long after its publication, our global economist team will be drawing on their proprietary data and other analytical resources to help Capital Economics clients stay on top of what this evolving technology means for their decision-making.
But our Spotlight project is an indispensable first step to meeting the many opportunities and challenges that artificial intelligence presents.
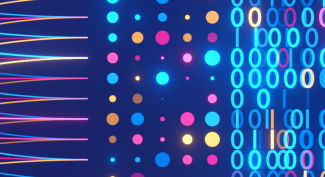
Part one: AI's economic revolution
If, as we believe, AI should be considered a general-purpose technology (GPT), then the implications for the macroeconomy could be huge.
There are generally considered to be three classic examples of GPTs, all of which had profound economic effects. The first took place during the Industrial Revolution with the development of the steam engine in the UK during the 1700s and 1800s. The second occurred with the introduction of electricity in the US in the early 1900s. And the most recent GPT wave took place during the late 1900s with the ICT digital revolution. Although it may not fit the definition on its own, the invention of the internal combustion engine in the late 1800s was, if not a fourth GPT, then at the very least a major technological innovation.
GPTs tend to affect economies in three phases. In the first phase, while the technology is still new and not widely used, the benefits to productivity are small. We discuss the reasons for the slow adoption of new technologies in more detail later in this section. An extreme example of this was during the Industrial Revolution in the UK, in a 50-year period known as “Engel’s Pause”, which was categorised as a time of strong innovation and capital accumulation but low productivity growth.
During the second phase, the technology is improved upon, the cost of implementation tends to fall and the technology becomes more widespread, eventually leading to significant productivity gains. Finally, in the third phase, the law of diminishing marginal returns takes effect. At this point the pace of improvements and rollouts slows, causing productivity gains to taper off.
What this means is that, historically, it has often taken decades for productivity gains from GPTs to materialise. However, this delay has been shortening. This is illustrated by the steady decline in “adoption lags”, namely the amount of time for countries to implement a new technology after it is invented. (See Chart 1.)
Chart 1: Technology Adoption Lags (Years) |
|
Source: Comin and Mestieri (2018) |
Chart 2 shows productivity gains from past major technological breakthroughs in those countries driving the progress. The boost to annual productivity growth in the UK and US from steam and electricity averaged a modest 0.2% or 0.3%, in part because, especially in the case of steam, the gains came through over such a long period. But the effect of the ICT revolution was bigger, in part because shorter impact lags meant that the gains were more compressed. The US, which saw far by the biggest gains from ICT developments, is estimated to have enjoyed an average boost to annual productivity growth between 1995 and 2005 of 1.5 ppts.
Chart 2: Contributions to Labour Productivity Growth (% Points Per Annum) |
|
Sources: Crafts (2020), Capital Economics |
We know that GPTs can have major implications for economic development, especially for those countries at the forefront of innovation. The fact that the US was at the forefront of major technological inventions such as electricity and the internal combustion engine in the early 1900s – and reaped the ensuing productivity gains – helped it to surpass the UK as the world’s biggest economy.
The invention of a GPT does not necessarily lead to strong productivity gains, though. Historically, the largest productivity gains have taken place in instances when technological advancements coincided with political and/or social changes which allowed the technologies to be exploited to their full potential. For example, it has been argued that Great Britain was able to lead the Industrial Revolution because it was better able to exploit its resources, including labour, thanks to the social and political reforms during the Enlightenment.
The impact of previous GPTs on labour markets has generally been positive. Major technological advances have caused significant short-term friction in the labour market. But workers have ultimately been able to transition to new higher paying jobs. This is because the GPT-related increase in productivity has either increased demand for certain goods or services or has created demand for new sectors altogether. A recent MIT report estimated that 60% of workers in the US are now employed in occupations that did not exist in 1940.
Unsurprisingly, the effects have not been homogenous across sectors. Workers have lost out in cases where a GPT has been a perfect substitute for their work, such as the agricultural workers during the Industrial Revolution who were replaced with automatic threshing machines. On the other hand, workers have generally benefited when a GPT has helped to augment the type of work they do.
Similarly, GPTs have led to the loss of some industries, but also the creation of entirely new ones. The invention of railroads eventually killed demand for horses, while electricity did the same for whale oil. But with the advent of railroads also came the need for workers to drive the trains, man the carriages and work at railway stations.
BOOSTING PRODUCTIVITY: THE EFFICIENCY GAINS
So, if past GPTs are anything to go by, the AI revolution will deliver huge changes across economies, including a big boost to productivity. In this section, we think through the channels through which this might occur and what we might expect in terms of the size and speed of productivity gains.
The most straightforward way in which AI will boost productivity is via one-off efficiency savings (by which we mean doing more with the existing resources or doing the same with fewer resources).
In some instances, these will be achieved by AI directly replacing humans because of AI doing more efficiently what humans currently do. In other instances, the savings will be achieved by AI helping humans to become more productive in their existing jobs, freeing up time to spend on other, potentially more productive, tasks. Moreover, AI could free up resources other than human capital; for example, the use of shared driverless vehicles would allow land used for parking to be utilised more productively.
Chart 3 helps to illustrate the channels through which these productivity gains might be achieved. In general, output per hour worked can be increased in three ways: i) raising the amount of capital per worker ii) raising the quality of the workforce and iii) raising multi-factor productivity i.e. the efficiency with which labour and capital are combined.
Deploying AI, which is basically a new form of capital, has some impact on productivity through raising the amount of capital per worker. This is most likely to be through the addition of new AI software, although AI might also slow the depreciation of non AI-capital by enabling more preventative maintenance.
But the bigger impact of AI is set to be the boost it gives to multi-factor productivity by, for example, facilitating better working practices. There are many examples of this with past technologies. When electricity replaced steam in factories, workers no longer had to be arranged around the centralised power source, enabling more effective production lines. The invention of the steam engine and the need to manage railroad schedules led to the adoption of standard time, helping people to use their time more effectively. And the development of email and other digital communications facilitated outsourcing and offshoring. An example of how AI might improve working practices is by using predicted demand to improve how firms determine and vary their staffing needs.
Chart 3: How Labour Productivity Growth Can Be Achieved |
|
Source: Capital Economics |
The potential efficiency gains resulting from all this are huge. Because AI is still at a relatively early stage, studies of its effects are scarce and, because the technology is developing so quickly, soon go out of date. Nonetheless, those that are available point to a significant boost. For example, an April 2023 study of call centres, showed that access to AI assistance increased the productivity of agents, as measured by the number of customer issues they resolve per hour, by 14%.
Admittedly, in some cases, the application of AI is a zero-sum game and, while boosting productivity for some firms at an individual level, will not do anything to help at the aggregate level. This includes the use of AI in targeted online advertisements and in the automated trading of financial instruments.
Equally, though, it is easy to imagine the deployment of AI in some sectors having a far bigger positive impact. For example, in the time previously taken by a lawyer to draw up and check one contract, they might be able to manage ten contracts which had been provisionally drawn up by AI. There have been countless studies that crunch laboriously through each type of job, classifying what share of each could potentially be automated by AI and with what effect. But there is so much uncertainty that precise estimates are meaningless. A simple back-of-the-envelope calculation based on some plausible assumptions makes the point that this could have major implications for productivity across a wide range of job type.
BOOSTING PRODUCTIVITY: AIDING INNOVATION
A more uncertain, yet potentially even more important, way in which AI might boost productivity is if it helps to further technological progress. This could occur if it serves as a new general purpose “method of invention”. For example, the greatest impact of optical lenses was to aid R&D via microscopes and telescopes, rather than helping people see better.
AI could sift through the vast amount of research already published to search out further research avenues. There are literally millions of scientific papers published each year and there is no way that human researchers can digest everything in their field. But AI can, and it can look for potential relationships and suggest the most viable research streams. Indeed, one of AI’s advantages is thought to be its ability to follow unexpected threads and “think outside the box”, drawing researchers’ attention to patterns and connections which they might otherwise overlook. Meanwhile, AI can also help by using its knowledge to predict the results of real-world experiments.
AI has the potential to boost innovation across a very wide range of fields, from drug discovery to education to transport. Imagine all the potential applications of using AI in the field of material science, where it could choose the best new chemical compounds to solve real world problems. It could help to develop new pesticides for the agriculture sector; new corrosion-resistant metals for the transport sector; or compounds that absorb pollution to aid the green transition.
Using AI to boost innovation would not just give a one-off boost to the level of productivity; it could boost productivity growth permanently. We could even end up with a virtuous cycle whereby AI helped itself to improve at innovation.
Admittedly, none of this applies if the pessimists are right and there are simply no new good ideas left to be had. Economist Robert Gordon argues that there were only a few truly fundamental innovations (including instant communications and using power on a mass scale) and that these have now been made. If true, AI or no AI, the scope for further technological progress is limited.
More likely is that new ideas are still possible, but as science becomes increasingly complex, it requires more effort to find those ideas. The world can still make technological progress, but to do so it needs to increase research effort to offset the fall in research productivity. According to one NBER study, the US must double the amount of research effort every 13 years to offset the increased difficulty of finding new ideas. If this is true, then AI is still a powerful tool to aid innovation. But at least part of its power will lie merely in preventing productivity growth from slowing further as research productivity continues to wane.
But what if there were still some things that AI could not do? Explosive growth would still be possible if spending shifted towards the AI-intensive sectors in response to their fall in relative price (so-called “Baumol’s cost euphoria”). For this to occur, real spending would need to rise more rapidly than relative prices were falling, to push up the share of nominal spending in these areas.
More likely, though, is that the areas that AI was able to automate fully would not be perfect substitutes for those which it cannot. (Indeed, as technology has made manufactured goods cheaper, consumers have spent a greater share of their nominal income on low-productivity services.) So, as part of the economy became increasingly automated, the unautomated, less productive part of the economy would grow as a share of GDP, limiting overall growth. Growth would still get an initial boost from automation; but over time, it would slow as it became increasingly constrained by those parts of the economy that were essential yet hard to improve.
THE BARRIERS TO ADOPTION
In theory, then, AI has the potential to be a real game-changer for productivity growth. But potential gains are one thing, realised gains are quite another.
The productivity boost from past transformative technologies has generally been more drawn-out and less dramatic than might have been expected considering the importance of the inventions. And economists have been puzzling for years over why the digitalisation of the economy over the past couple of decades (including the advent of mobile internet, cloud computing and the Internet of Things) has been accompanied by such weak productivity growth. Nor is there any evidence yet of any boost to productivity growth from early-stage AI. Despite the recent fuss about ChatGPT, AI has been around for a while; it is almost a decade since reports began to predict an AI-related surge in productivity growth. Yet productivity growth, particularly in advanced economies, has been sluggish. Indeed, since pandemic-related distortions have washed out, productivity growth in G7 countries in the past couple of years has been below its average since 2005. (See Chart 4.)
Chart 4: G7 Output Per Worker (% y/y) |
|
Source: Refinitiv, Capital Economics |
One potential explanation is mismeasurement. There is certainly something in this. But research generally concludes that this accounts for only a small part of the productivity paradox.
An alternative explanation is that the full effects of GPTs, including AI, cannot be realised until waves of complementary innovations are developed. To make the most use of the Internet, for example, we also needed the development of cloud computing and big databases. To make the most use of AI, we will need other complementary innovations. One example already developed is computer vision, whereby computers analyse images and video, which can be used in applications from autonomous vehicles to medical diagnostics. There will be other complementary innovations which we cannot yet imagine.
The other key reason why technology does not translate into immediate productivity gains is that, even if the technology is available, many firms do not deploy it immediately or effectively. However, deploying AI is not just about plugging in some software and letting it do its thing. Companies need the accompanying capabilities, such as databases, data management systems and IT specialists.
Moreover, making the most of a new technology – AI included – requires significant organisational and process changes to make full use of the accompanying capital investments. For example, adapting to online selling was not just about setting up a website; retailers had to change all their logistics to include new warehouses, delivery channels etc.
Indeed, most of the factors that have slowed the exploitation of productivity gains from previous technological steps forward are just as applicable now as in the past. These barriers can be broken down into those that are internal (and within a firm’s control) and those that are external (which a firm cannot directly influence).
Of the internal barriers, the most important for many firms will simply be the costs required, especially in the near term when they will be highest. Indeed, a key uncertainty regarding the speed of adoption of AI is how quickly costs fall.
As for external barriers, regulation/regulatory uncertainty is likely to be key. Governments may place limits on how AI is deployed in practice. And firms may face, or fear facing in the future, costs associated with data security and privacy regulation.
The upshot is that the pace of AI deployment is not simply dependent on technical feasibility; instead, it will depend on various factors including costs, regulatory barriers and the skills of the workforce. Policy will also play an important role in all of this. Indeed, AI is most likely to deliver a big boost to productivity if several factors come together, including a rise in investment, reskilling of the workforce, and a balanced regulatory regime.
QUANTIFYING THE BOOST
So where does this leave us? While AI’s potential benefits are large, a “big bang” of productivity gains seems unlikely; it would be more realistic to expect any boost to come through gradually, as in the past.
We think that the impact of past technological advances is the best guide to the likely impact of AI in the coming years and, given the nature of AI, the ICT revolution is probably the best one to look at. Data show productivity gains during the ICT revolution reached 1.5% per annum for the US, so that seems like a reasonable guide to what is achievable for a country that is at the forefront of developing and deploying the technology. It is even possible that the gains from AI are bigger than this; whereas many applications of the digital revolution were about improving the consumer experience, we think that AI has many more business use cases that will directly boost productivity.
One thing to note about the ICT revolution, however, is that the US was by far the biggest beneficiary in terms of productivity gains. The largest euro-zone economies, for example, saw little boost, while some other countries (including the UK, Canada and Australia) were in-between – experiencing some productivity uplift, but not on the scale of the US. This reflects differences in the policy environment as well as economic structures that affected the adoption of new technologies.
The lesson is that productivity gains from AI are by no means guaranteed and will depend on whether countries have the factors in place to help them utilise AI effectively. Below, we explore in more detail the extent to which countries away from the technological frontier will benefit from AI.
CONCLUSIONS
AI is far enough advanced, with sufficient business cases, that we can be confident that there will be a significant productivity boost at some point. However, while AI’s adoption lags may be shorter than for previous technologies that required significant replacement of physical capital, there are still various reasons why its adoption is likely to be slow and fitful. As with past technologies, the productivity boost is therefore likely to be more of a slow-burn than a miraculous surge. Any productivity boost is more likely to be a late 2020s and 2030s story than something to be expected over the next few years.
To the extent that the past GPTs are a good guide to what to expect, we might expect a boost to annual productivity growth in countries which successfully and fully deploy AI of 1.5% for a period of a decade or two. However, not all of this will necessarily be picked up in the official GDP figures. And the extent to which countries successfully utilise AI, and therefore benefit from productivity gains, will differ significantly.
Jump to the section "Blowing bubbles? AI's financial market implications"
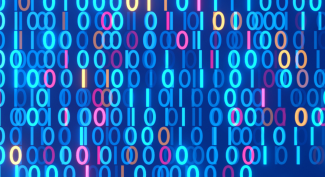
Part two: AI and the global economic order
INTRODUCING THE AI ECONOMIC IMPACT INDEX
AI has the potential to be a game-changer for productivity growth, but a host of factors will determine whether countries can reap the benefits. We argued above that the effects of new technologies cannot be realised until waves of complementary innovations are developed. And even if the technology is available, the extent of productivity gains will depend on the ability of policymakers and firms to diffuse it effectively. Finally, once the technology is deployed, economies need to be able to adapt so that displaced workers and capital can be redeployed.
With this in mind, our new, proprietary AI Economic Impact Index provides a tool for assessing which economies (a mix of 33 major DMs and EMs) are best placed to benefit from AI and its effects over the next couple of decades. The index uses 40 sub-indicators to construct three pillars covering the three factors that influence the impact of AI – innovation, diffusion and adaptation – which then provide a composite score. Clients of CE Advance can access the data behind the pillars and the composite index here.
Chart 5: AI Economic Impact Index |
|
Source: Capital Economics |
THE US LEADS THE WAY
The US ranks first. This suggests that it will once again assume the role of the global technological leader as it has done for much of the past 100 or so years. This reflects its size, the rise in both private and public investment in R&D, and the rapid expansion of the higher education system.
Our index shows the US well ahead of the pack in innovation. Much of this reflects the dynamism of its private sector. The US has, by a long distance, channelled more private investment into AI than anywhere else. It also has the largest number of AI start-ups. This isn’t solely a reflection of its size; the US also fares well on these measures in per capita terms.
CHINA MAY STRUGGLE TO TAKE ADVANTAGE
China also scores well on innovation due to the significant resources the public and private sectors have channelled towards AI since it was identified as a national priority in the mid-2010s. But China scores lower on diffusion. Regulators have insisted on much greater oversight of the generative AI tools developed by Chinese firms than has been seen elsewhere, in large part to ensure they operate within censorship rules. We also suspect that the leadership’s efforts to direct the activities of China’s firms – evident in the crackdown on the tech sector – will make economy-wide adaptation to the new technology harder. Accordingly, while China leads the way in some areas of innovation, it only ranks 18th in our index – the highest of any major EM, but a middling performance overall.
Despite that, China could still play a significant role in how the AI landscape develops globally. As happened with internet services, China is walling off its domestic market and nurturing an independent ecosystem of AI providers. But unlike in the early years of the Internet, China may now be able to export successfully some of these services.
ASIAN TIGERS AND THE UK WELL-PLACED TO BENEFIT
Hong Kong, Singapore and South Korea rank highly. Singapore is in second place, with Korea and Hong Kong also in the top 10. These economies perform well on diffusion and adaptation in particular. Indeed, a feature of their export-led development and subsequent move up the value chain has been their ability to adapt quickly to new technology. For example, Korea was able to replicate technology from Japan when developing its heavy industry in the 1970s and repeated the trick with the growth of the digital TV market in the 2000s.
The UK comes third. This may come as a surprise given its perennially low investment rate. But set against that, the UK has a world-class higher education system that draws in top talent from around the world. As a result, it has a particularly high ranking in our Index for AI innovation. Global AI-leader Google DeepMind is based in London. The UK’s economy is more services-oriented than most others too, lending itself to the relatively quick diffusion of AI. And its flexible labour market should help the wider economy adapt to the challenges and opportunities presented by AI.
JAPAN AND EURO-ZONE PLAYING CATCH-UP
Japan and most euro-zone economies sit somewhere between the US and China in our ranking. Japan (16th place) does have a strong track record in technological innovation and is facing the same demographic drag as the Asian Tigers, which may increase the speed of AI adoption. But it has a poor recent record in diffusing new technology across the economy. For example, cash is still used more widely in Japan than in any other major rich economy. Government surveys show that the vast majority of businesses still use fax machines.
There are several constraints facing major economies in the euro-zone. It’s notable that, in stark contrast to the US (and to a lesser extent the UK), the euro-zone did not receive a productivity boost from the ICT revolution in the 1990s/early 2000s. It’s likely that several other factors – including German reunification and euro-zone monetary union – overwhelmed the impact of ICT on productivity. But weaker productivity growth was also because the US played a bigger role in driving the technological advances and therefore got a bigger boost from the expansion of the ICT sector. History may be repeating itself in that regard. Indeed, Europe’s less developed venture capital industry and its relatively poor cloud infrastructure – a critical component in the development of AI – does not bode well for its innovation potential.
What’s more, a combination of familiar structural problems is also likely to hold back the diffusion of AI, at least relative to the US. In the 1990s/2000s, European companies were generally smaller and less able to achieve economies of scale from IT. Labour markets were less flexible, making it harder to reorganise the workplace to take advantage of new technologies. Local planning restrictions and regulations were onerous too (and slowed the move to “big box” retailing). Many of these issues persist.
There are differences between ICT and AI. But these differences may make Europe even less well placed to benefit from AI than from ICT. For example, European policymakers may be more likely to subject AI to regulation than policymakers in other countries.
Of course, the euro-zone itself isn’t a homogenous bloc; some countries will do better than others. Countries with workforces that have higher levels of AI-related skills and/or greater network readiness (Germany, France) are better placed to adapt than those with fewer AI skills and low network readiness (Italy, Spain). (See Chart 6.)
Chart 6: Network Readiness and STEM Graduates |
|
Sources: Portulans Institute, UNESCO, Capital Economics |
AI IN EMERGING ECONOMIES – A BLESSING OR CURSE?
So far, our discussion has been focussed on economies that are typically regarded as being at, or close to, the technological frontier. But what does AI mean for convergence (or “catch-up” growth) between those at the frontier and those further away from it – typically emerging economies?
In general, EMs – particularly those outside Asia – rank significantly lower than DMs. At the risk of oversimplifying, low dynamism in the private sector, small and less-advanced IT sectors, relatively low R&D investment, and the brain-drain of top talent to richer economies will prevent EMs from being technological innovators as well as slow the diffusion of technology relative to richer economies.
Chart 7: CE AI Economic Impact Index Composite Scores (out of 100) |
|
Source: Capital Economics |
LLMS A THREAT TO SERVICES-LED EM DEVELOPMENT…
There are other points to explore in the EM context. Our forecasts are underpinned by the belief that generative AI, in particular LLMs, has the potential to revolutionise how tasks are performed in certain services. A ubiquitous example of this is the proliferation of chatbots in customer services. But there are others too, such as drafting legal contracts or producing personalised marketing emails. Many of these tasks are outsourced to low-income EMs (hence the term “business process outsourcing” or BPO sector). These areas could see substantial productivity increases.
But they tend to be formulaic and repetitive, such that AI can replace rather than complement humans. The risk is that Western firms paying for BPO services increasingly decide to do them in the cloud. There is a dearth of research, but a study from the World Bank and University of Oxford on customer service firms in India found that “AI adoption initially coincides with a small increase in general hiring, but then reduces demand for non-AI workers over the next few years, such that the overall effect is substantially negative”.
India, as well as the Philippines, are the posterchildren for the BPO sector. Their comparative advantage lies in having low labour costs as well as large pools of workers who are literate in English. So what scale of damage could AI do to these economies? The first point is that the BPO industry is smaller than often assumed. It accounts for around 0.4% of total employment in India and 1.5% in the Philippines. (See Table 1.) At 6.5-7%, the share of GDP is larger, but not enormous.
Table 1: BPO Sectors |
|
Sources: Various, Capital Economics |
In both countries, BPO sectors have grown faster than other parts of the economy over the past couple of decades. In an extreme scenario where the BPO sector got completely wiped out over the next decade and there were no redeployment of capital or labour to other sectors of the economy, the direct hit to GDP growth would be around 0.8%-pts per year in both countries. All else equal, that would imply a drop in potential growth to about 5.2% in India and around 4.5% in the Philippines. While detrimental to income convergence, economic growth would remain relatively robust.
In any case, redeploying capital from the BPO sector wouldn’t be that difficult (call centres could be repurposed for other offices or housing, while ICT is used in lots of sectors). And as for labour that might be displaced, there are opportunities to reskill (or upskill). Demand for AI-related services – for example in training or sense-checking output – may grow, a lot of which can be outsourced. That was another finding of the World Bank/University of Oxford study.
To be clear, reskilling or upskilling won’t be an option for all displaced workers. It requires training and education (i.e. human capital) which is expensive, and most low-income EMs are fiscally constrained. But it does at least offer one method to mitigate the potentially damaging effects of AI on employment.
With all of this in mind, we think that the BPO sector could enter a slow demise that knocks around 0.3% to 0.4%-pts per year off GDP growth in India and the Philippines over the next decade. Again, not helpful, but not a disaster. Other countries that would be affected by shrinking BPO sectors are Brazil, Mexico and Poland, though not to the same extent as their Asian counterparts. A hit to GDP of around 0.1-0.2% per year seems a reasonable estimate.
…BUT AI APPLICATIONS CAN PLUG DEVELOPMENT GAPS
Viewed more optimistically, like other technological advances before it, AI may offer a chance for low-income EMs to gain widescale access to services that have a material economic impact. A recent example is the mobile phone revolution that swept sub-Saharan Africa in the late 2000s, though this technological “leapfrogging” appears less applicable in the case of AI (after all, it is not superseding an existing technology). But the bigger point is that the mobile phone revolution brought undeniable benefits which could offer lessons for AI adoption.
It is feasible that, as with the mobile phone revolution, a handful of EMs will be very quick to implement AI technology. Some of the barriers to AI adoption in richer economies won’t exist in very poor economies. There may be greater social acceptance of AI if it is perceived as aiding development. For example, in the absence of enough skilled professionals, AI may be able to fill the skills gap in things like medical diagnostics.
To reiterate, there are factors that will prevent the majority of EMs from deploying AI on a wide scale in the near future. And it’s important to stress that having greater access to services is no guarantee of income convergence. AI won’t be able to solve – among other factors – the problems of weak institutions, poor governance and lack of regional integration that tend to hold back development in poorer economies.
But this shouldn’t detract from the potentially transformational impact of AI – maybe even larger than mobile phones – in many other areas. The use cases in medicine and healthcare mentioned above could bring huge benefits in countries that suffer from, for example, high infant mortality rates. AI also has the potential to address the skills gap in education.
WHAT DOES ALL OF THIS MEAN FOR PRODUCTIVITY?
So how, then, will all of this shape the global economy over the coming decades? Taken as a whole, productivity growth in the G7 could rise back towards the rates seen during the ICT revolution of the 1990s that preceded the slowdown in the 2000s and 2010s. That said, this would still be weaker than the 3% to 4% rates of productivity growth seen in most advanced economies in the 1950s and 1960s, which was underpinned by specific factors such as industrialisation, rapid growth in trade and catch-up from the Great Depression and Second World War.
What’s more, there will be significant differences between countries. We forecast that productivity growth in the US will average 2.3% a year in the 2030s – similar to the rate that was recorded during the 1990s ICT boom. Productivity growth elsewhere will be lower. We forecast that it will average around 1.5% a year in the 2030s in Canada and the UK. In the case of the UK this is well above the rates of the past two decades but some way below the rates recorded in the 1990s. Productivity growth will also rise in Japan, France and Germany but to around rates of 1.0-1.5%, reflecting their lower scores on our AI Economic Impact Index. And in Italy, we think that not even the AI revolution will be able to lift productivity growth above 1%.
Chart 8: CE Forecasts for G7 Output Per Worker (%, y/y, Annual Average) |
|
Sources: Refinitiv, Capital Economics |
AI will also deliver a productivity boost to China. But in contrast to DMs, we do not anticipate a pick-up in overall productivity growth compared to previous decades. This is because other structural headwinds pushing in the opposite direction will overwhelm the impact of AI. Slower diffusion of AI across the economy means that most EMs will receive a productivity boost later than DMs, perhaps from the mid-2030s onwards. Prior to that, productivity growth in EMs such as India that have relatively large services exports sectors could be slower than otherwise if those sectors get re-shored back to richer economies, though these sectors aren’t big enough for their gradual decline to cause massive shocks to output.
THE WORLD ECONOMY AFTER THE AI REVOLUTION
All of this has implications for the shape of the global economy in the wake of the AI revolution.
If we are right that AI will boost advanced economies more than emerging economies, this will amplify a shift in the global trajectory that’s been underway for a while: a slowdown in the rate at which average EM incomes are catching up with developed-world incomes, compared to the "EM Golden Age" of the 2000s and early-2010s.
Similarly, for the world’s two largest economies, AI is likely to help the US economy sustain its primacy over China in terms of GDP measured at market exchange rates. The AI revolution is another reason to think that expectations of China’s economy streaking ahead of the US will have to be further reined back.
By contrast, India will move up the rankings, from the world’s fifth largest economy currently to its third. But for the next decade at least AI will hamper rather than help its rise.
All told, the potential for AI to deliver a much-needed boost to productivity growth in advanced economies means that they are likely to account for six of the ten largest economies in 2040. (See Chart 9.) The fact that these economies – plus, we think, India – are all likely to remain aligned geopolitically with the US points to another implication: The AI revolution is likely to entrench the dominance of the US and its allies across the global economy and add to the difficulties that China will face in trying to both compete at the technological frontier and to reduce its dependence on critical inputs from the West.
Chart 9: CE World Rankings by Nominal GDP at Market Exchange Rates |
|
Source: Capital Economics |
CONCLUSIONS
Three broad conclusions can be drawn from all this. First, our proprietary AI Economic Impact Index suggests that the US will lead the AI revolution, with the Asian Tigers, UK, Israel, and parts of the Nordics also well-placed to benefit. Second, China will lead some aspects of the AI revolution but struggle in others. This is in part because AI is likely to become a new fault line in the fracturing of the global economy. Third, the AI revolution will make EM income convergence harder as richer economies are better equipped to deploy the technology on a wide scale.
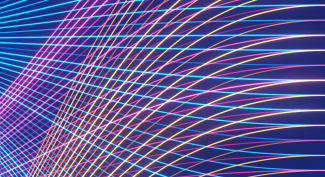
Part three: Blowing bubbles? AI's financial market implications
AI COULD BRING HIGHER REAL RATES AND BOND YIELDS
Many of the effects of AI that we have identified in our analysis will have implications for equilibrium real interest rates in the major economies. While it is by no means clear-cut, our judgement is that the net effect of these factors will be to put some upward pressure on those rates.
One reason is that the equilibrium real interest rate in an economy is positively related to its potential real rate of economic growth, which we expect to be boosted by productivity-enhancing investments in AI.
The equilibrium real interest rate in an economy is also positively related to the profit share (the share of income flowing to the owners of capital), which we suspect will rise from already high levels.
What’s more, the equilibrium interest rate in an economy is negatively related to the overall savings rate, which might fall in response to expectations of higher future income amid the prospect of faster, AI-fuelled economic growth.
Admittedly, savings rates could arguably rise, rather than fall, if – as suggested above – AI boosted the share of income flowing to the owners of capital at the expense of the share flowing to labour. And they could also rise if AI complemented, and therefore benefited, skilled labour by more than unskilled labour (i.e., “skill-biased technological change”). This is because those groups tend to have a lower marginal propensity to consume.
Nonetheless, while we acknowledge that there is a high degree of uncertainty surrounding the net effect of AI on the determinants of equilibrium interest rates, we still think the most likely outcome is that, all else being equal, it will push them up.
This would be similar to previous technological revolutions: historical estimates from the Bank of England show long-run real interest rates generally rose in the aftermath of technological breakthroughs, as the benefits of the new discoveries slowly diffused through the economy. (See Chart 10.) (Although in the case of the Internet, this relationship was overshadowed by other contemporaneous factors, such as the Global Financial Crisis (GFC).)
Chart 10: Global GDP-Weighted Real Interest Rate (25y MA, %) |
|
Sources: Schmelzing (2020), Refinitiv, Capital Economics |
We also expect real sovereign bond yields to remain generally above their average level over the past decade, given that they are mainly a function of expectations for real short-term interest rates.
Of course, AI is just one of many factors that affect equilibrium rates, which we are exploring in a separate piece of work to be published in October 2023. As it happens, we believe some of the other key factors – including the effects of the green transition and ageing populations – will have a similar impact to AI.
On the other hand, AI could have a disinflationary impact in the short term, in a similar fashion to the increase in the global supply of labour following China’s integration into the world trading system. This could help to offset upward pressure on nominal bond yields from any rise in real yields.
The upshot is that we think equilibrium rates and “safe” bond yields will be higher than in the pre-pandemic decade, but not dramatically so, and that the increase will be gradual. We suspect that the US 10-year Treasury yield will rise from an average of 2.4% in the 2010s to around 4.0-4.5% in the 2030s, with the real yield rising to around 1.5-2.0%.
EQUITIES STAND TO BENEFIT FROM AI'S ADVENT...
Admittedly, higher "risk-free" bond yields would put downward pressure on the valuation of equity markets, all else equal. However, we expect this to be more than offset by faster growth in corporate earnings over time.
Whether corporate earnings will grow even faster than output in the domestic economy will depend on two things. The first is whether the corporate sector’s share of output rises. The second is whether corporate earnings grow faster than corporate output (i.e. the share of income flowing to company earnings increases).
The former seems unlikely – notwithstanding some cyclical fluctuations, the share of GDP accounted for by the corporate sector has been fairly consistent over time. However, it is possible that the share of output flowing to company earnings could increase from what is already a high level by past standards. (This is the flip side of a lower labour share.) In this instance, corporate earnings would grow at a faster rate than overall output, resulting in faster growth in stock prices, all else equal.
…BUT THEY WON'T BENEFIT EQUALLY
In the near term, we think the boost to equities will be concentrated among the providers of AI, principally located in the tech sector. Indeed, it is this group of firms who drove the S&P 500 higher in the first half of this year, as hype around AI outweighed fears about slowing economic growth. (See Chart 11.)
Chart 11: Total Return Indices (End-2022 = 100) |
|
Sources: Refinitiv, Capital Economics |
We believe various factors will determine which user industries will be the fastest to adopt AI. Industries in which tasks are typically more repetitive could, for example, automate roles within the workplace using AI models most quickly, benefitting from the greater efficiency and precision it affords. Likewise, industries whose operations involve the use of large datasets may be quicker to realise the benefits of using AI to assist with more laborious tasks.
By contrast, we think the industries which will be slowest to adopt AI are those in which tasks typically involve manual labour which cannot be automated, such as construction, as well as those for whom the risks from using AI pose the biggest threat. This could be due to risks to security or potential threats to customer safety.
However, one of the most important themes of this report is that AI should be regarded as a general- purpose technology. That is to say, it has multiple applications, which will eventually affect all sectors of the economy. As such, although these industries may be slower to adopt AI as they are likely to see less direct benefit from utilising the technology, they are still likely to benefit indirectly as the gains from its adoption broaden out across the economy.
There is also bound to be some discrepancy in relative equity performance at a regional level.
Among developed markets, we think the US is set to be the star performer. Indeed, although it is by no means the only economy in the process of developing the technology, an overwhelming share of the firms at the heart of recent enthusiasm around AI is listed on US exchanges. This is hardly surprising. The US’ dominance of consumer tech markets in recent decades has spawned hubs such as Silicon Valley to which hordes of AI start-ups have been attracted, and often been acquired by nearby tech giants. Indeed, the number of newly funded AI companies in the US has far exceeded that in any other country over the past decade.
We are already seeing this head start in innovation beginning to play out in equity markets, establishing the US as a frontrunner.
Other DMs look set to lag the US for various reasons. Whilst Japan has a remarkable track record for innovation, the country scores poorly on our adoption measure given its slow uptake of modern technologies in recent decades. That said, firms dealing in machinery and electronic equipment account for almost 30% of the firms listed in the four major AI ETFs. Given that a quarter of these industrial firms are listed in Japan, the country could benefit if robotics were to receive a boost from the AI rally.
Meanwhile, we think many euro-zone countries could suffer from the region’s relatively poor cloud infrastructure – a critical component in the development and deployment of AI – as well as strict regulation, hampering its ability to innovate and deploy the technology.
Instead, we think the greatest threat to the US’ dominance looks likely to come from some other East Asian economies. In China, we believe the ability of the state to mobilise resources rapidly could support AI-based innovation in the next few years. That said, China’s poor prospects for diffusion of AI technology and application throughout the economy could mean any positive impact on equity markets is limited to the tech sector. Meanwhile, the Asian Tigers (Hong Kong, Singapore, Korea and Taiwan) rank highly on our AI Economic Impact Index, given their histories of harnessing new technology relatively quickly, as well as Taiwan’s status at the forefront of semiconductor production. We believe this could help the region’s equity markets outperform others – especially given the large weighting of tech sectors in some of these countries’ indices.
IS HYPE AROUND AI CREATING A BUBBLE?
The release of several high-profile generative AI applications last year has caused quite a stir among investors, in presenting some of the most tangible evidence of the potential capabilities of AI to date. Indeed, stock prices of firms specialising in AI software and semiconductor production have rallied strongly since the launch of OpenAI’s ChatGPT in November 2022, with tech giants Nvidia and Meta performing particularly well. (See Chart 12.)
Chart 12: Share Prices (3rd Jan. 2023 = 100) |
|
Sources: Refinitiv, Capital Economics |
This has come at a time when the rest of the stock market has performed relatively poorly given concerns around slowing economic growth. (See Chart 12 again.) Indeed, only a little over a quarter of the firms in the S&P 500 have risen in price by more than the overall index this year – the lowest proportion on record. (See Chart 13.)
Chart 13: Share of S&P 500 Constituents Which Have Outperformed Overall Index (%) |
|
Sources: Refinitiv, Capital Economics |
Such a sharp but narrow rally raises the question of whether a speculative bubble may be forming in the stock market.
We are of that opinion. Clearly AI has the potential to be a transformative technology which revolutionises how goods and services are produced and provided to consumers. But the extent of the current euphoria suggests that investors are wanting to crystallise the potential financial gains from the technology upfront.
This is not entirely dissimilar to what happened in the late 1990s, when stock prices rose as excitment built around the possibilities of the internet. Indeed, the last time gains in the S&P 500 were as narrow as they currently are was during this so-called “dot-com bubble”. (See Chart 13 again.)
Nonetheless, it is worth remembering that, while the share prices of some companies may once again appear extremely high in relation to their past earnings per share (EPS), shareholders have a claim on the future EPS of a company. And the faster EPS are expected to grow, the higher the relative price/trailing 12m (TTM) earnings ratio of its shares will be vis-à-vis their relative price/forward earnings ratio.
Imagine, for example, that Company A and Company B both have TTM EPS of $10. They trade at share prices of $1000 and $100, with price/TTM earnings ratios of 100 and 10, respectively. The EPS of Company A are expected to double each year for the next four years, while those of Company B are expected to grow by only 12.5% each year during the same period. On this basis, the price/forward four-year (F4Y) EPS ratios of Company A and B will be at, or around, 6.25. In other words, the current valuation of Company A’s share price doesn’t appear so heady when measured against analyst expectations for what the company will earn in the coming years.
Back in the real world, we can see the effect if we compare the price/earnings ratios of Nvidia with those of the S&P 500 and a selection of other big tech firms. While Nvidia is an outlier based on TTM EPS, it doesn’t really look like one based on analysts’ projections for EPS in four years’ time. (See Chart 14)
Chart 14: Price/Earning Ratios |
|
Sources: Refinitiv, I/B/E/S, Capital Economics (including estimates) |
The flipside is Nvidia’s EPS are forecast to grow more rapidly than those of the other firms. (See Chart 15.)
Chart 15: Latest & Analyst Forecast EPS (TTM EPS* = 100) |
|
Sources: Refinitiv, I/B/E/S, Capital Economics (including estimates) |
HOW MIGHT A BUBBLE EVOLVE?
In our view, the formation, and ultimate bursting, of a bubble in AI would probably have four key features.
First, we think it would mainly be a US phenomenon. As discussed earlier, investors appear to believe that it is primarily US-based tech firms which are best placed to benefit from the adoption of generative AI. By contrast, European equities are receiving a limited AI-related boost given the region is home to few large tech companies (SAP and ASML are notable exceptions).
This could significantly hamper the ability of the region’s stock markets to keep pace with the US stock market in the next couple of years, given that it was the success of large-cap tech and telecom stocks such as Nokia and Ericsson which helped European equities to narrow the gap with their US counterparts at times during the dot-com bubble.
This will particularly be the case if the US’ “pull factor” entices foreign firms to relocate, such as UK-based semiconductor firm, Arm.
Second, a bubble would probably take years to play out, and result in a stronger stock market overall.
The dot-com bubble lasted at least five years, with then-Fed Chairman Alan Greenspan warning about “irrational exuberance” in the stock market almost four years before it burst.
Admittedly, valuations were lower in the mid-1990s than they are now, suggesting we may already be further along in the process. High-frequency trading and the increased role of social media in influencing market moves also mean that markets tend to move faster these days than they used to. This is evidenced by an increase in the number of “mini-bubbles”, with recent examples including biotech and cryptocurrencies. But we still expect a bubble to last for at least two more years. And we expect the stock market in general to rally, as the bubble inflates, like it did during the second half of the 1990s.
All sectors of the S&P 500 performed well during the second half of that decade, even if the information technology sector outperformed massively. In our opinion, the AI revolution is likely to be similar: our 2024 and end-2025 forecasts for the S&P500 are 5,500 and 6,500 respectively.
Of course, the adverse effect of tight monetary policy on economic growth could prevent a bubble from inflating. After all, history shows that equities have nearly always fared poorly in downturns. (See Chart 16.) And information technology was the worst-performing sector on average in the last three US recessions (not counting the COVID-induced one).
A period of slower growth could therefore cause enthusiasm around AI to wane temporarily. However, we envisage that this would take some air out of a bubble rather than causing one to burst. A shift towards policy easing by the Fed and other major central banks would also help to create a favourable environment for a bubble to reflate subsequently.
Chart 16: US Equities (S&P 500/Composite) & GDP (Real, Log. Scale, Jan. 1950 = 100) |
|
Sources: Refinitiv, Shiller, MeasuingWorth, NBER, Capital Economics |
And even if an economic downturn is more severe than we foresee, stock markets have tended to ride out recessions when there has been excitement around a prospective new technology. The dot-com bubble is not a useful point of comparison here, given the US economy did not enter a downturn until after the bubble had burst. But further back in time, railroad equities – another former bubble in the stock market – powered ahead in the 1860s and 70s, despite persistent weakness in the economy.
Third, while we are not opining on the outlook for Nvidia’s shares, a couple of examples from the dot com era suggest what could conceivably happen to touted “winners” in the stock market after a bubble eventually burst. The examples reveal that the valuations of these winners came off the boil well before expectations for their EPS started to decline; the slide in their valuations was sufficiently large to cause their share prices to drop very sharply; and it took a long time for their valuations to recover (if at all), even after their EPS had done so.
The first example is Cisco. It was widely seen as integral to the success of the worldwide web, and the company’s price/TTM earnings ratio (based on last reported TTM EPS) maxed out at above 200 in the spring of 2000. Once the bubble burst, however, its valuation tumbled and trended down until the GFC, since when it hasn’t really recovered. The upshot is that Cisco’s share price is virtually unchanged since the end of 1999, despite some growth in its EPS. (See Chart 17.)
Chart 17: Cisco's Share Price, P/E Ratio, & EPS |
|
Sources: Refinitiv, I/B/E/S, Capital Economics (including estimates) |
The second example is Microsoft. While its price/TTM earnings ratio at the end of 1999 was above the market average, it was less than Cisco’s – partly reflecting an expectation that its earnings wouldn’t grow quite so rapidly. After the bubble burst, Microsoft’s lofty valuation also fell back and trended down until the GFC, dragging its share price lower. Unlike Cisco’s, Microsoft’s valuation has rebounded sharply since then. And the percentage gain in its earnings has been larger since the end of 1999, with the result that its shares have racked up a big gain. (See Chart 18.) Nonetheless, the fact remains that the turnaround in Microsoft’s share price after the dot com bubble burst took many years.
Chart 18: Microsoft's Share Price, P/E Ratio, & EPS |
|
Sources: Refinitiv, I/B/E/S, Capital Economics (including estimates) |
Fourth, we would not expect the bursting of a bubble to sound the death knell for AI, any more than the bursting of the dot-com bubble sounded one for the Internet. This is because we expect AI, like the Internet, to have lasting implications for firms in many industries.
CONCLUSION
We expect AI to be a productivity-enhancing technology, resulting in a modest and gradual rise in equilibrium real interest rates, in turn keeping real sovereign bond yields generally above their average level over the past decade.
Although higher equilibrium real interest rates could weigh on the valuation of equity markets, we still expect equities to benefit from the adoption of AI as faster growth in the economy boosts growth in corporate earnings. We think these gains will be seen in most industries given the widespread application of generative AI, although firms developing AI-focussed software and hardware look set to benefit most in our view – particularly in the near term. At a regional level, we think the US stock market is poised to be a frontrunner given it is home to many of these firms.
Tighter monetary policy and slower growth may be a headwind for US equities in the next few months. But we don’t expect that to last, as central banks shift towards policy easing and economic growth picks up in 2024, allowing an AI-fuelled bubble to reflate.